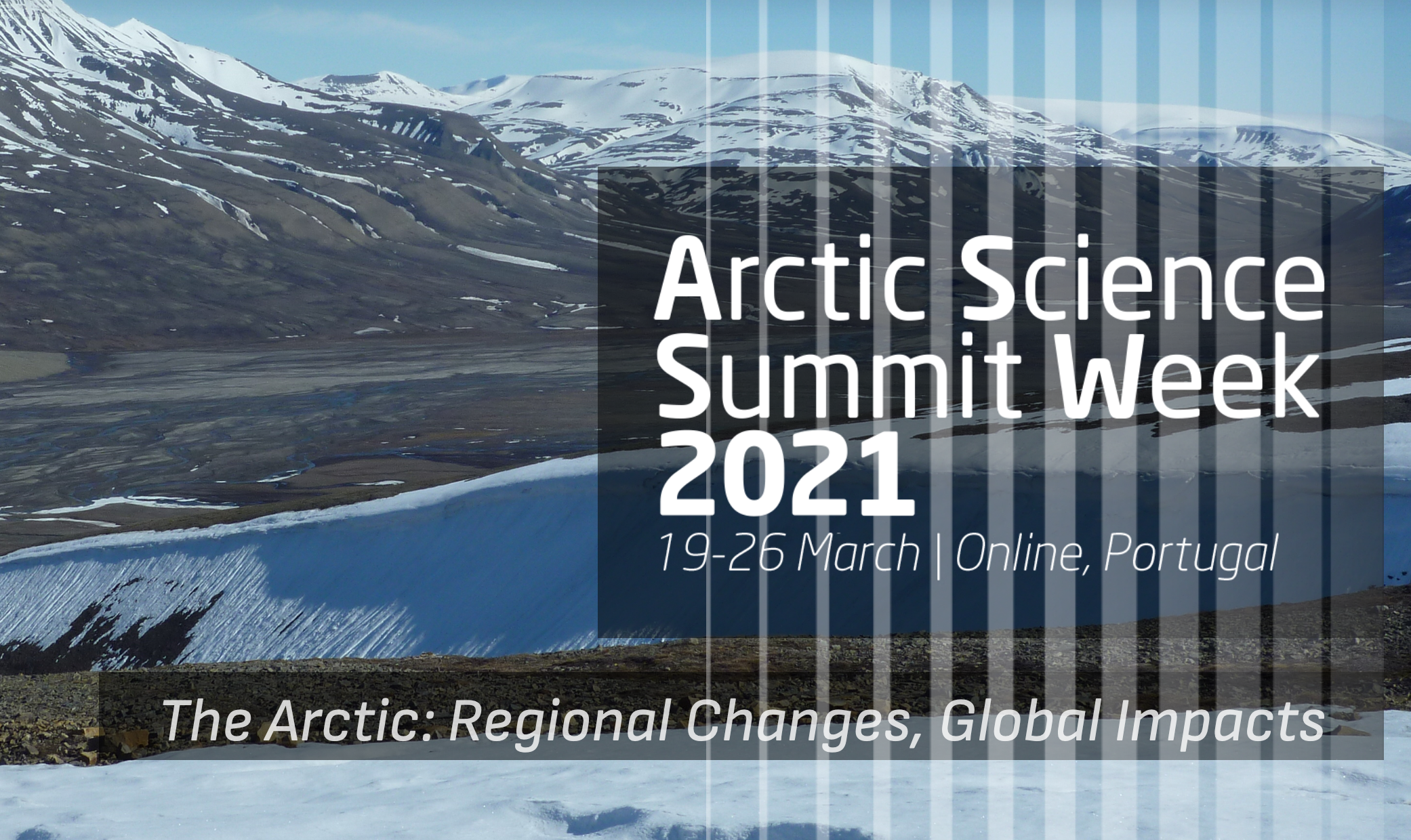
APPLICATE at ASSW21
15. March 2021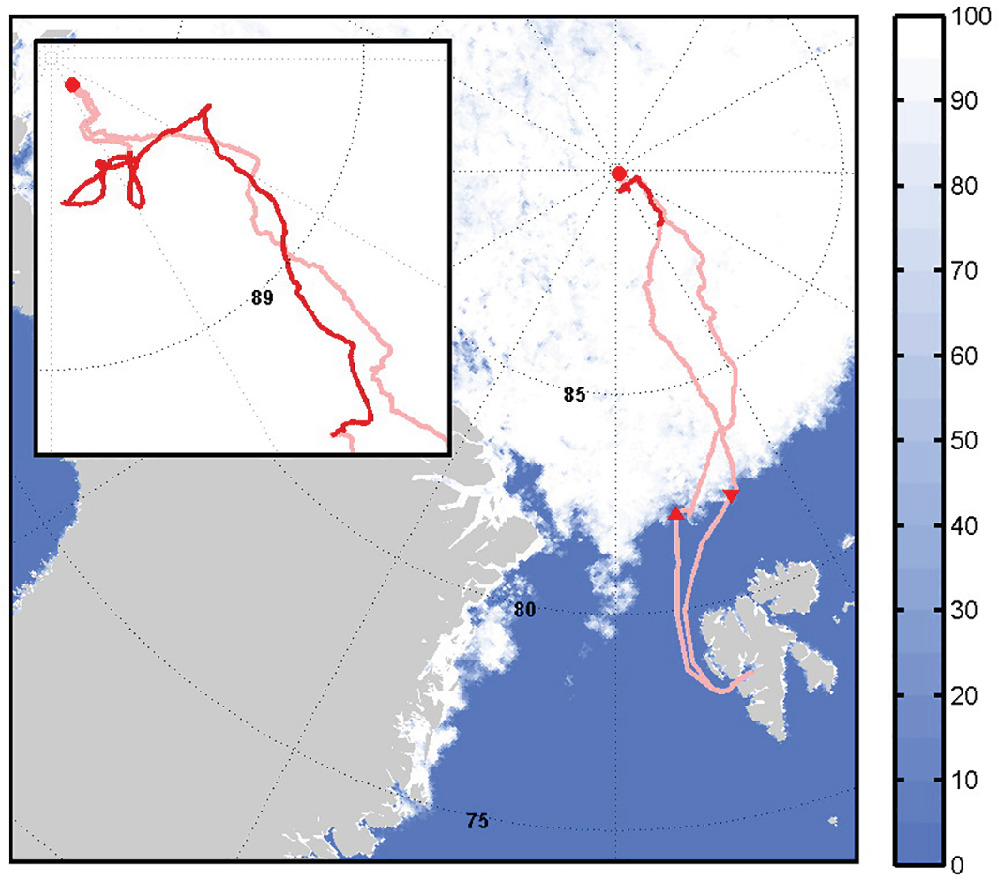
Central Arctic weather forecasting: Confronting the ECMWF IFS with observations from the Arctic Ocean 2018 expedition
7. April 2021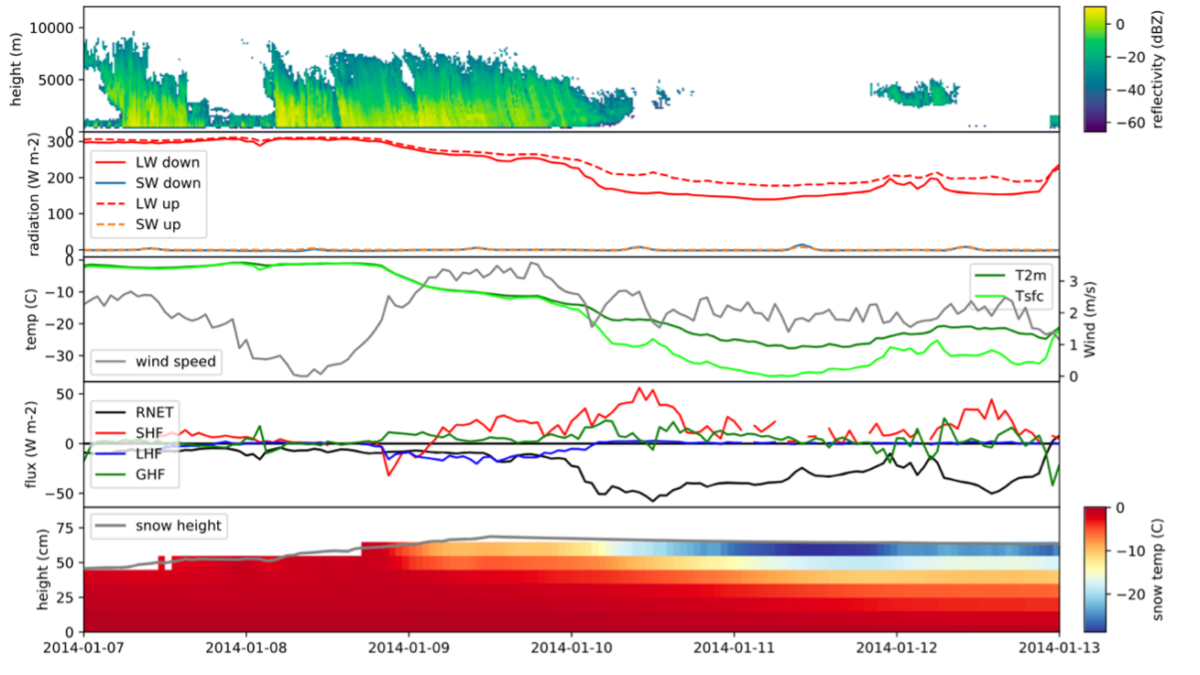
By Daniel Butkaitis (AWI), Jonny Day (ECMWF), Mayleen Schlund (AWI)
In the polar regions predictions of near-surface (2m) air temperature and other variables within the atmospheric boundary layer, are marked by large systematic errors. Many processes have to be taken into account for accurate forecasts of near-surface air temperature, such as turbulent mixing, radiation and cloud microphysics. As errors in these different processes can compensate each other, it is a challenge to make sure forecast skill is improved due to better process representation, and not as a result of changing the balance between compensating errors. The dominant paradigm to identify the sources of forecast error in the past has been numerical experimentation, but diagnostic-focused analyses can provide additional insight and inform the model development process.
In a new article, Jonathan Day et al. from the European Centre for Medium-Range Weather Forecasts (ECMWF) present a set of process-oriented diagnostics (PODs) to assess the response of surface temperature to radiative forcing in Earth System Models. These PODs have been used to analyze the impact of a new multi-layer snow scheme in ECMWF´s Integrated Forecast System (IFS). In a previous study, Arduini et al. (2019) concluded that this new multi-layer model reduced the bias in T2m and snow depth overall. With the POD analysis, it is now possible to confirm that these forecast improvements are happening for the right reasons.
Compared to the single-layer (SL) snow scheme, the multi-layer (ML) snow scheme can represent the vertical structure of a snowpack (e.g., temperature and density). Figure 1 shows observational results and displays the importance of vertical structures of snowpacks in Sodankylä, Finland. The number and thickness of active snow layers are computed diagnostically at the beginning of each model time step. Depending on the snow depth, one to five layers are then used. Compared to the SL model, liquid water content is computed prognostically instead of diagnostically.
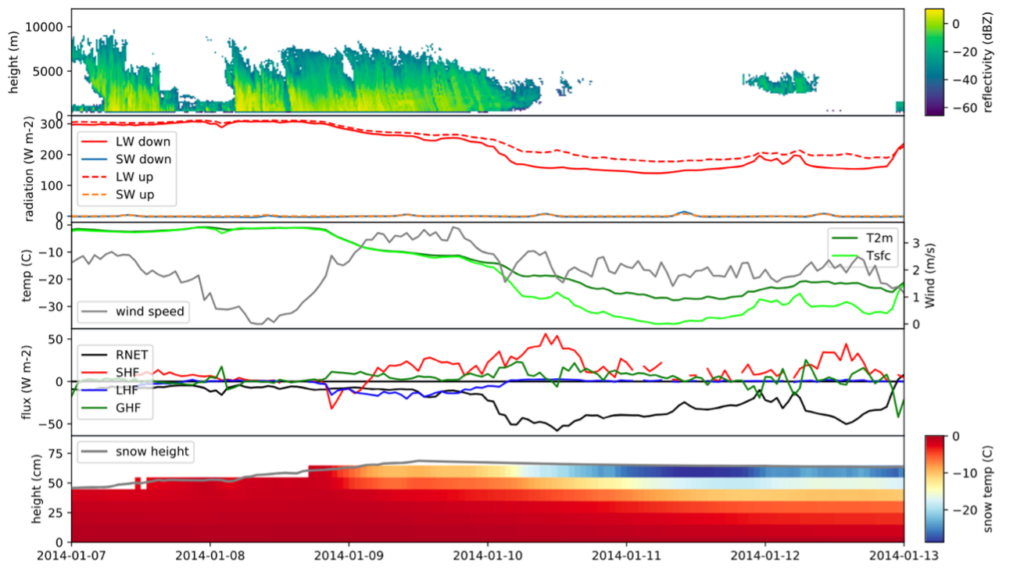
Figure 1. Observed meteogram for a case study at Sodankylä, Finland, in January 2014. It shows (from top‐to‐bottom) cloud radar reflectivity (from CloudNet; Illingworth et al. (2007)); radiation terms; wind speed, surface, and 2 m temperature; energy balance terms: Total net radiation (RNET), sensible (SHF), latent (LHF) and ground
(GHF: Atmosphere snow) heat flux (with the sign convention that terms are positive when directed at the surface); and snow temperature at various heights (above the soil‐snow interface).
For the model evaluation, data from two supersites has been used: Sodanklyä, Finland and Summit, Greenland, which are located in different climate zones. The winter 2013-2014 period was chosen, as measurements of all Surface Energy Budget (SEB) components are available for this period at both sites. The PODs used in this study are based on those presented by Miller et al. (2018). In this approach, the SEB is separated into a single “driving term” and multiple “response terms”. As the authors state, this method has the “desirable property that deficiencies in the behavior of the SEB can be diagnosed in isolation without the confounding effects of other sources of error”.
This novel diagnostic approach of dividing systematic errors in 2 m temperatures into errors in radiative forcing and errors in boundary-layer and land-surface processes has to date only been applied over snow covered surfaces. However, in the future, the methods will also be applied to understand model error and evaluate model developments in lower latitudes where errors in the diurnal cycle, for example, are acute.
To read the full paper: Day, J. J., Arduini, G., Sandu, I., Magnusson, L., Beljaars, A., Balsamo, G., et al. (2020). Measuring the impactof a new snow model using surface energy budget process relationships. Journal of Advances in Modeling Earth Systems, 12, e2020MS002144. https://doi.org/10.1029/2020MS002144